It was my freshman year, 1991. I was enthusiastic to finally be learning about biology, after being forced to waste a year in the German army’s compulsory service at the time. Little did I know that it was the same year a research paper was published that would guide the direction of my career to this day, more than 30 years later. Many of the links in this post will go to old web pages I created while learning about this research.
The paper in question contained two experiments that seemed similar at first, but later proved dramatically different. The first one was conceptually most simple: a single Drosophila fruit fly, tethered at a torque meter that measures the force a fly exerts as it attempts to rotate around its vertical body axis (i.e., trying to turn left or right), controls a punishing heat source. For instance, attempting to turn to the left switches the heat on and attempting to turn to the right switches the heat off. There is a video describing the way this experiment was set up at the time:
In the paper, my later mentors Reinhard Wolf and Martin Heisenberg described how the flies learn to switch the heat on and off themselves and how they, even after the heat is permanently switched off, maintain a tendency to prefer the turning directions that were not associated with the heat. The “yaw torque learning” experiment was born. Quite obviously, yaw torque learning is an operant conditioning paradigm, as the fly is in full control of the heat.
In another experiment in the same paper, the flies control the angular position of a set of black patterns around them with their turning attempts, pretty much like in a flight simulator (also described in the video above): whenever the fly attempts to turn, say, left, the computer rotates the patterns to the right, giving the fly the visual impression of actually having turned to the left. There are two pairs of alternating patterns arranged around the fly and one set is associated with the same punishing heat beam as in yaw torque learning, such that the fly can learn to avoid flight directions towards these patterns in this “visual pattern learning” experiment.
Like yaw torque learning, visual pattern learning appears to be an operant experiment, as the fly controls all stimuli. However, this conclusion may be premature, as the flies may just learn that one of the patterns is associated with heat, just as the Pavlovian dog learns that the bell is associated with food. Wolf and Heisenberg addressed this question by recording the sequence of pattern rotations and heat applications from one set of flies and playing it back to a set of naive flies. If the Pavlovian association of patterns with heat in the “replay” (or ‘yoked’) control experiment alone was sufficient to induce the conditioned pattern preference, the operant behavior of the flies would just be a by-product of an essentially Pavlovian experiment. However, there was no preference for a pattern in the “replay” flies, so visual pattern learning in the Drosophila Fight Simulator is still an operant experiment at its core – despite the conspicuous ‘contamination’ with a Pavlovian pattern-heat contingency.
In the course of my early studies, I was entirely oblivious of this research, until in 1994 I took a course in Drosophila Neurogenetics where I learned about these two experiments. I remembered Pavlov’s classical conditioning experiments from High School, as well as operant conditioning in Skinner boxes. Both Pavlov and Skinner having been dead for some time, I thought the biological differences between operant and classical conditioning must be well known by 1994, so I asked Reinhard Wolf during the course, if he could explain to us the biological differences between operant and classical conditioning: what genes were involved and in what neurons? To my surprise, he answered that nobody knew. He said there was some genetic data on classical conditioning and some neurons in a brain area called “mushroom bodies”, but for operant learning, nobody knew any biological process: no genes, no neurons, nothing. I was hooked! One “nobody knows” reply to a naive undergraduate question was all it took to get me set up for life. I felt that this was a fascinating research question! What are the neurobiological mechanisms of operant learning and are they any different from those of classical learning?
The following year I started working towards answering this question in my Diploma thesis (Master’s thesis equivalent). It seemed to me that to be able to tackle that question, I first needed to understand what “the operant” actually was, so I could study its mechanisms. To get closer to such an understanding, I collected a large dataset of 100 flies in each one of four experimental groups: One group was trained in visual pattern learning as described above. Another group was trained in a Pavlovian way, such that the patterns were slowly rotated around the fly such that each pattern would take 3 seconds to pass in front of the fly, such that the heat would be on for three seconds with one pattern in front of the fly and 3 seconds heat-off when the other pattern was passing before the fly. The two remaining groups received the same treatment as the two first groups, but without any heat. Both classical and operant experiments were set up such that the pattern preference after training, tested without heat, was of about the same magnitude. To achieve this, the classical experiment had to be set up that the flies received multiples of the amount of heat that the flies in the operant experiment would receive (i.e., the 3s heat-on/3s heat-off procedure). I wondered why that had to be this way? Why did operant training require much less heat than classical? I hypothesized that the operant flies may learn specific behaviors that get them out of the heat quickly or that enable them to avoid the ‘hot’ patterns more efficiently. To test this hypothesis, I fine-combed the behavior of the flies with a myriad of different analyses I coded in Turbo Pascal – to no avail. I could not find any differences in the behavior of the flies that would explain why the operant flies needed so much less heat than the classical ones. Despite there being two differences in the classical and the operant setups, i.e., more heat and no operant control in the classical experiments, there didn’t seem to be any major difference in the animals’ behavior. Obviously, I may just have missed the right behavioral strategy, but lacking any further ideas where or how to look for them, I cautiously concluded that the operant flies may somehow learn their conditioned pattern preference more efficiently when they are allowed to explore the patterns with their behavior, as opposed to slower learning when the patterns were just passively perceived – some kind of “learning by doing” maybe? Heisenberg’s pithy comment from those days is still stuck in my mind: “This is a genuine result, but at the same time, the world is full of non-Elephants.”
Despite the negative results, I enjoyed this type of research enough and found the research question so exciting that I wanted to continue in this direction. I decided to do my PhD in the same lab with Martin Heisenberg as my advisor and I was lucky he had a position available for me, so I started right after I had handed in my Diploma thesis in 1996.
In my ensuing PhD thesis, I tried to further come to grips with the fact that operant visual learning seemed to be so much more efficient than classical visual learning. My first approach was to eliminate one of the two differences in my previous Diploma work, the amount of heat the classically training animals received. I wanted the only difference between the experiments to be “the operant”, i.e., the operant control over the stimuli. I started by turning towards the “replay” experiment, where the flies passively perceive the same pattern/heat sequence that was sufficient for the active, operant flies to learn their conditioned pattern preference. But in this experiment, the passive, “replay” flies (i.e., the classically trained ones) did not show a preference, so I couldn’t really compare them with the operant flies that did show a preference. Why did the “replay” flies not learn? After all, the patterns were associated with the heat and this association was sufficient for the operant flies to learn. It turned out that by doubling the “replay” training, the “replay” flies started to show some preference, but much weaker than the operant flies. In this experiment, the only difference between the two groups of flies is the operant control, everything else is exactly identical. Together with the data from my Diploma thesis, this prompted the hypothesis that the animals may really just be learning that one pattern is “bad” (i.e., associated with heat) and the other “good”, irrespective of whether the animals learned this operantly or classically. The only difference between the operant and the classical experiment seemed to be that operant was much more effective than classical, but in all other aspects, there didn’t seem to be a difference between operant and classical learning. Could it be possible that at the heart of operant visual learning lies just a genuinely Pavlovian association between pattern and heat?
One of the hallmarks of a truly Pavlovian preference is that classically conditioned animals are able to express their preference of, in our case the ‘cold’ pattern, with any behavior, e.g., they should approach the ‘cold’ pattern and avoid the ‘hot’ whether they are, say, walking or flying. After much fiddling around with the setup (with the help of the mechanical and electronics workshops!), it turned out that to test this hypothesis, for technical reasons, I needed to combine the yaw torque experiment with the pattern learning experiment and replace the patterns with colors. The outcome was an experiment in which the flies controlled both colors and heat via their left/right choices, e.g., left turning yielded blue color and heat off, while right turning leads to green color and heat on. “Switch-mode learning” was the informal name for this procedure. Very long story short: it turned out that there really is a genuinely Pavlovian association formed in such switch-mode learning. Flies that learn that, e.g., green is good and blue is bad, can avoid the bad color and prefer the good color in a different behavior than the one that they used to operantly control the colors with. This means that there may be a fundamental difference between the operant yaw torque learning and operant visual learning: In the operant visual experiment, the operant behavior is important, but it does not play any role in what is being learned, only how. It doesn’t seem to enter into any association at all. Instead, it just seems to facilitate the formation of an essentially Pavlovian stimulus-stimulus association. In contrast, in the yaw torque learning experiment, there isn’t anything else that the animals could possibly learn, but their own behavior. In a way, yaw torque learning is a ‘pure’ form of operant learning, while operant visual (i.e., pattern/color) learning is ‘contaminated’ with a very dominant Pavlovian component. Both are operant experiments, but what the flies are learning looked likely to be dramatically different. Would that difference also affect the biology underlying these learning processes?
In the light of these results, I concluded my thesis work with a study on some classic (pardon the pun) Pavlovian phenomena. I had mixed feelings towards my achievements so far. On the one hand it felt like I got a bit closer to understanding the commonalities and differences between operant and classical learning, but I certainly hadn’t been able to find any genes or neurons involved in operant learning. Some reviewers of the work emphasized this shortcoming.
After graduating in the year 2000, I moved for my postdoc to Houston, Texas to study another form of ‘pure’ operant conditioning in an animal where we could get access more easily to the neurons that are involved in the learning process, the marine slug Aplysia. There I learned how the biochemical properties of a neuron, important for deciding which behavior will be generated, change during operant learning and how this leads to more of the rewarded behavior. This work was like a booster to my initial curiosity about the biological mechanisms of operant learning. More than ever before I felt that it now was high time for new approaches to discover which genes work in which neurons in Drosophila operant learning.
As mentioned above, for Drosophila classical conditioning, several learning mutants had been isolated decades earlier. After moving from Texas to Berlin in 2004, we tested them in our “switch-mode” operant color learning experiment. Consistent with the idea developed in my PhD work that such operant visual learning is essentially just an operantly facilitated Pavlovian learning task, some of these mutants are also defective in operant visual learning – likely because the Pavlovian ‘contamination’ is so dominant, as the previous experiments had suggested. How would these mutants fare if we took the colors away such that the ‘contaminated’ “switch-mode” became ‘purely operant’ yaw torque learning? To my surprise, the mutant flies did really well! The first of these experiments weren’t actually done by me, even though they had been on my to-do list for years by then, but by an undergraduate student – I only replicated them a few months later. These results made it unambiguously clear that there really was more than a conceptual difference between operant yaw torque learning and operant stimulus learning: there was a very solid biological difference. If there was a stimulus to be learned, i.e., a “Pavlovian contamination”, then Pavlovian learning genes were involved, but once that contamination was removed, Pavlovian learning mutants did just fine in the resulting ‘pure’ operant learning task. While this work was done with the mutant rutabaga, which affects a cAMP synthesizing enzyme, the results from a different gene were even more surprising: flies where the function of the protein kinases of the “C” type (protein kinase C or PKC) were inhibited, behaved in exactly the opposite way: they did fine in visual learning but failed in the ‘purely operant’ yaw torque learning task. This work took four years and in 2008 we published that we had found a gene family specific for operant learning, PKC.
So by that time, some 14 years later, I had a first answer for the initial question that got me started in the first place: there is a biological difference between operant and classical learning and you only see it if you remove all “classical contamination” from the operant experiment. Now that we had a gene family, what we needed next was one or more (as many as possible, really) individual genes and the neurons they are expressed in. It turned out to be quite difficult to find out which of the six PKC genes in Drosophila is the one important for yaw torque learning. Julien Colomb, a postdoc who had joined me in Berlin, used both mutants and RNAi approaches but was only able to rule some of the PKCs out, but did not find the crucial one. Things looked a bit better on the front where we tried to identify the neurons: Whichever PKC it was, it was apparently important in motor neurons. That may not sound so odd, after all, we are conditioning a behavior and motor neurons control the muscles for the behaviors. But these motor neurons were located in the ventral nerve cord (the “spine” of the insects) and we had thought operant conditioning was something that needed to involve the brain. So while the results were rather clear, I nevertheless didn’t value them sufficiently, as I was convinced the brain was more important than the ventral nerve cord, no matter what these experiments tried to tell me. There probably was a good explanation for these results, I thought then, once we find out what is really happening in the brain. The results were what they were and we published them in 2016.
While these experiments were ongoing, another candidate gene had appeared on the horizon, the transcription factor FoxP. The background for this candidate goes back to 1957 and the book “Verbal Behavior” by BF Skinner. In the book, Skinner claimed that the way we learn language looked an awful lot like operant learning: trying out phonemes until auditory feedback tells our vocal system that we have indeed said the words we wanted to say. This seemed like rats trying out behaviors in a Skinner box until they have found out how to press the lever for food. Or, I thought, how flies try out behaviors until they have found out how to control the heat with their yaw torque. While these all really did seem to look very analogous on the face of it, this view was shredded already in 1963, in a book review by Noam Chomsky, then a young scholar. But this critique not only helped catapult Chomsky to fame, it was also one of the starting points of what later was called the “Cognitive Revolution”. One of Chomsky’s most hard-hitting arguments was that the analogy was simply a false analogy and that Skinner had not provided any real evidence at all. A few years before his death, Skinner acknowledged the criticism and agreed he did not have any evidence other than the observed superficial parallels. In 2001, a gene was identified that would bring this classic academic feud back into public focus. A mutation in the human transcription factor FOXP2 was identified as the cause for a very specific speech and articulation impairment, verbal dyspraxia. A few years later, one of my colleagues in Berlin, Constance Scharff and her team knocked down the same gene in their zebrafinches and got an analogous phenotype: the birds had trouble learning their song. By then I was electrified! If Skinner had been right and Chomsky wrong, then there was a chance that FoxP really may be an operant learning gene. In 2007, I asked Troy Zars (who died way to soon some years later) at a meeting if he knew whether flies had a FoxP gene at all. It turned out he already had a set of mutants in his lab and was willing to collaborate with us on this project. Within a few weeks of the mutants arriving, the data started to emerge that we finally had found a single gene involved in yaw torque learning – and it couldn’t have been a more Skinnerian gene! In operant visual learning, the mutants did fine, just like the flies with the inhibited PKCs. After some additional experiments to make sure the results were solid, the work was published in 2014. It really started to look as if yaw torque learning shared more than just a conceptual similarity with vocal learning in vertebrates. It now appears the biological process underlying these forms of learning evolved in the last common ancestor of vertebrates and insects, some 500 million years ago.
Our next experiments started to capitalize on this discovery. In 2012, I had become a professor in Regensburg and a few years later, one of my grants on this research question got funded. So we hired two PhD students to help me. One of them, Ottavia Palazzo, created a suite of genetic tools manipulating the FoxP gene and the neurons where it was expressed. Among other things, it turned out that FoxP was also expressed in motor neurons in the ventral nerve cord, the neurons where PKC was required for yaw torque learning. The work started in late 2017 and in the following year, a sabotage case, where a (likely mentally ill) postdoc in the lab kept damaging (and eventually destroyed) some of our equipment, brought most of these experiments on operant learning to a screeching halt. Soon after we had unmasked and fired the saboteur (which took the better part of another year), the Covid19 pandemic started, making everything even tougher on everyone. We managed to publish the work we had done with FoxP in December 2020. By that time, the gene scissors CRISPR/Cas9 had become really useful for genetic manipulations. Ottavia Palazzo had already used them to create the FoxP tools and the second graduate student on the grant, Andreas Ehweiner, now “CRISPRed” some PKC genes we hadn’t been able to properly test, yet, and struck gold. It turned out, the atypical PKC (aPKC) was the PKC gene we had been looking for all these years! If you knocked aPKC out in FoxP neurons, yaw torque learning was severely impaired. So now we had two individual genes involved in operant learning.
This was a strong indication that FoxP and aPKC may act in the same neurons for yaw torque learning to work. A quick analysis of the expression patterns of the two genes suggested overlap in a specific set of motor neurons in the ventral nerve cord, namely the ones that control the angles of the wings to, e.g., create left- or right-turning yaw torque, the “steering motor neurons“. At the same time, we could not find any overlap between aPKC and FoxP in the brain at all, suggesting that the neurons we were looking for definitely did not reside inside the brain. Some other of Andreas’ experiments also seemed to confirm that. Now all of the evidence we had pointed away from the brain and to the steering motor neurons in the ventral nerve cord. So was it really them? To quantify which of these neurons actually expressed both aPKC and FoxP, collaborator and motor system expert Carsten Duch in Mainz, painstakingly dissected all the tiny muscles that are attached to the wing hinges and then analyzed which of the individual motor neuron terminals on the muscles showed the markers for both genes. He discovered that it really was just a very circumscribed subset of the steering motor neurons that expressed both genes and not all of them. Specifically, the neurons involved in generating the slow torque fluctuations were were conditioning in our yaw torque learning experiment were the ones expressing both genes and those involved in, e.g., fast body saccades, or thrust or roll, did not express both genes. All of this pointed to these specific neurons, but so far, it was only circumstantial evidence. What we needed to do now was to find some way to check if the aPKC/Foxp-dependent plasticity, as we suspected, was really taking place in these steering neurons and not by some bad luck in some neurons we hadn’t on our radar. It was 2023, and genetic lines that would allow us to target just the specific steering motor neurons for slow torque behavior and none of the others were just in the process of being generated, so there really wasn’t a perfect way for a genetic experiment just yet.
So we tried to come up with some other way to get more data on whether all the evidence that was pointing towards these neurons really was sufficient, or if there was some alternative explanation for our data that we hadn’t thought of. It hadn’t escaped our notice that the function of the motor neurons we were eyeing had been described in the context of optomotor responses. The optomotor response is an orienting behavior evoked by whole-field visual motion. Its algorithmic properties entail that the direction of the whole-field coherent motion dictates the direction of the behavioral output (e.g., leftward visual stimuli lead to turning left, and rightward visual stimuli lead to turning right). The currently available evidence suggests that this visual information, in flies, gets transmitted directly from the brain to the steering motor neurons via a set of descending neurons in the brain. With the brain being ruled out as a site of aPKC/FoxP-dependent plasticity, the steering neurons were the only conceivable overlap between optomotor responses and yaw torque learning. This meant that if yaw torque learning altered the steering neurons in some way, we may be able to detect this plasticity by some change in the optomotor response of the trained flies. Or, phrased differently, if we were to detect effects of yaw torque learning in the optomotor response after operant training, this would be very strong evidence that the plasticity we were looking for indeed was taking place in the steering motor neurons.
We tested this hypothesis by comparing optomotor responses before and after yaw torque learning. We found that the optomotor responses to visual stimuli that elicit responses in the turning direction previously not associated with the heat did not change. However, responses to stimuli that elicit optomotor responses in the punished direction were selectively reduced. The figure below shows the torque traces after training, separated into those flies that were punished on left-turning (yellow) and those punished on right-turning. Reference, untrained amplitudes are just over 500 units of torque, as one could guess from the respective unpunished directions in each group. Always the torque amplitude of the punished direction is reduced, i.e., right (clockwise) rotations elicit reduced torque in flies punished for right turning attempts (green), compared to the same responses in flies punished for left-turning attempts (yellow) and vice versa for counter-clockwise rotations.
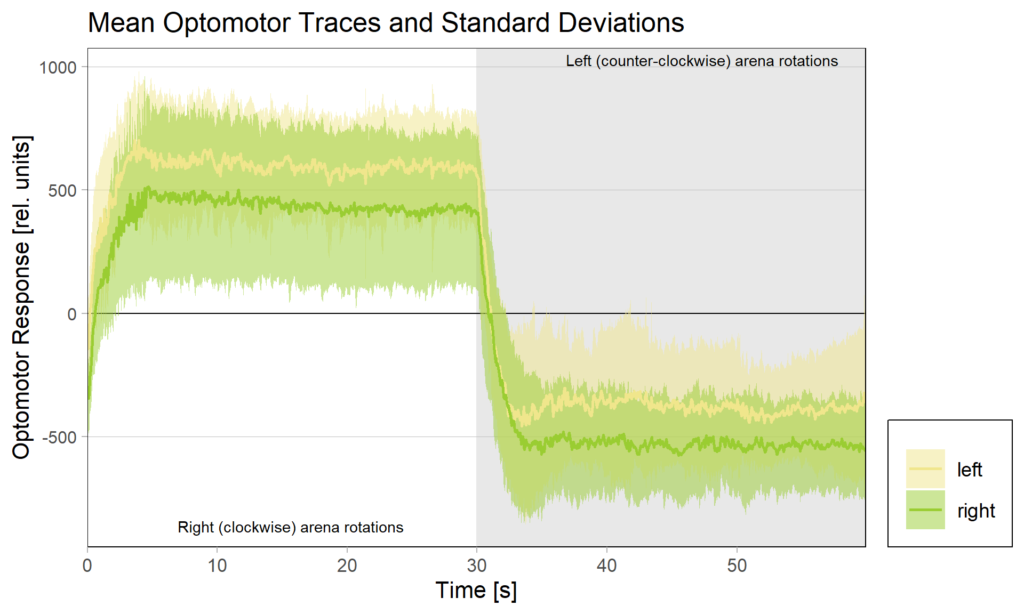
These results showed that indeed the plasticity seems to happen in the motor neurons that innervate the steering muscles generating large torque fluctuations – so pretty much at the very last stage. The changes in optomotor responses after torque learning only explain about 30% of the variance in the torque data. This means that there are flies that show, e.g., strong preference for the unpunished turning direction, but only a weak reduction in optomotor response on that side, as well as flies that, say, show a strong reduction in optomotor response, but only a weak preference for the unpunished turning direction. This means that there are very likely additional mechanisms at play, but, at least for now, these mechanisms do not seem to depend on aPKC/FoxP. As of now, this seems difficult to reconcile with the observation that knocking out any one of aPKC or FoxP completely abolishes yaw torque learning to undetectable levels, but this is what future research is for.
Today, almost exactly 30 years after a seemingly simple question when I was an undergraduate, we are finally in the process of localizing mechanisms of plasticity for “Skinnerian”, operant learning to specific neurons and specific genes. Now we can finally begin to really exploit the vast toolbox of Drosophila neurogenetics on a much larger scale than before to find the remaining parts of the puzzle: Which other genes are involved in this pathway? What are the biochemical and physiological changes in which parts of the neurons that give rise to the behavioral changes? How does yaw torque learning interact with visual learning to make it happen faster – aka the “learning by doing” effect? Why do “classical” learning mutants learn yaw torque better? How is this “Skinnerian” learning regulated, such that it is faster under some conditions (like when there are no colors present, i.e., pure) and slower under others (i.e., when the experiment is “contaminated” with Pavlovian colors)?
On the one hand, it seems the future has now become more boring, because it is so clear what experiments have to come next. In the past, I never knew what experiments to do until the current experiment was done. Too much was unknown, too few conceptual principles understood. Now, one just needs to open the Drosophila toolbox and there are enough experiments jumping at you for the next decade. On the other hand, the future has never been more exciting: I never have felt that the future promised any major advances – it was all too uncertain. Now that we have the first genes and the first neurons, it feels like the sky is the limit and that the next research questions will be answered dramatically more quickly than ever before.
Only now, looking back 30 years after having started from essentially zero, it is dawning on me that, building on the early behavioral experiments of Martin Heisenberg, we have now managed to open up a tiny little research field with a huge potential. The genes we found indicate that the biological processes we study is at least 500 million years old and present in most animals, including humans. It appears to be involved not only in language learning, but also more generally in many other forms of motor learning. And there are few other preparations around anywhere, in which these processes can be studied both in such splendid isolation and how they interact with other learning processes as in, e.g., habit formation. The only problem is, I likely won’t have another 30 years to capitalize on the efforts of the last 30 years: mandatory retirement in Germany will hit me in just 15 years from now. Humans surely live too short for the speed of this kind of research.